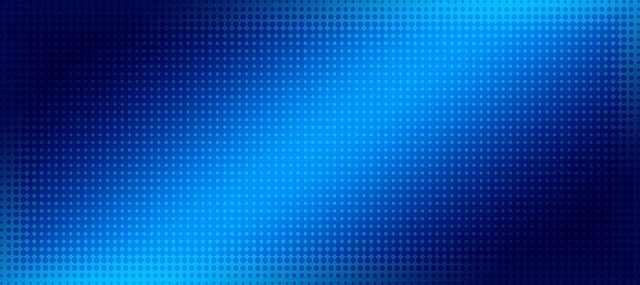
"Evolution of the Red Sequence in the CLASH Clusters" and others
ul. Pasteura 7, sala 404 / https://www.gotomeet.me/NCBJmeetings/seminarium-astrofizyczne
22 Oct, 2024 - 22 Oct, 2024
Seria: Seminarium Astrofizyczne
Prelegent i afiliacja: Aidan Cotter,Nicola Principi,Paweł Siegmund,Antonio Vanzanella - NCBJ, Warsaw
Data: wtorek 22 października 2024; 12:30
Miejsce: ul. Pasteura 7, sala 404 / https:meet.goto.com/NCBJmeetings/seminarium-astrofizyczne
Streszczenie:
Evolution of the Red Sequence in the CLASH Clusters Late type galaxies in cluster environments experience rapid quenching, evidenced by an excess of Red Sequence (RS) dwarf galaxies in low redshift clusters compared to more distant clusters. In this talk I will discuss how photometric data from CLASH was employed to quantify the evolution of cluster populations. The algorithms developed in this project provide a method for analysing cluster populations, the timescale of star formation quenching in clusters and probing the formation of early type galaxies.
Constraining the impact of redshift interlopers on the two-point and three-point correlation functions Since the early 2000s, the study of the Large Scale Structure (LSS) of the Universe has become central to cosmology. The European Space Agency (ESA) Euclid mission promises to significantly advance this field by delivering the most extensive and precise galaxy map to date in optical and infrared bands.
In this study, we assess for the first time the impact of interlopers in the measurement of cosmological parameters with the 3PCF. To do that, we derive and test a new expression for the 3PCF estimator that effectively disentangles the cosmological signal from systematic errors. We analyze the impact of redshift interlopers on the 2PCF and 3PCF signals and we model them, comparing pure samples (without systematics) to those contaminated by interlopers.
This work represents the first step in a full self-consistent treatment of realistic data considering in detail systematic effects as expected from the Euclid mission, demonstrating that addressing interloper contamination biases is essential.
Previous research experience: 'Analysis of the light response of a scintillation detector ' and 'Testing physics at the fundamental level with extragalactic sources' During my bachelor and master studies, I took part in several internal internship programs, one of it was called “Analysis of the light response of a scintillation detector” as part of the “Nuclear physics in research and its applications” research team. My master’s thesis, in turn, was written on a topic related to cosmology, it was titled “Testing physics at the fundamental level with extragalactic sources” and included calculations for testing gravity models using data from galaxy clusters. I would like to briefly tell you about my current scientific experience, focusing primarily on the elements mentioned above.
Detection of slow-moving objects using LSST Our objective is to develop a Machine Learning pipeline capable of identifying slow-moving objects (SMOs) present in the LSST image. The Legacy survey in space and time will commence in 2025, spanning a decade, and will provide an unparalleled catalog of celestial objects from the southern hemisphere. Faint and distant objects may pose significant challenges for the current automated pipeline, necessitating the development of our automated pipeline to detect SMOs such as long-period comets. We used a Machine Learning approach, specifically a three-dimensional Convolutional Neural Network. As the survey has yet to commence, we have used simulated data, provided by the Dark Energy Science Collaboration (DESC), where we have introduced simulated SMOs to create a Dataset. The simulated SMOs have been generated using the ephemeris of Trans-Neptunian objects obtained from the Jet Propulsion Laboratory and scaled to greater perihelion distances. Following this, we have partitioned the Dataset into Training, Validation, and Test sets, leveraging the first and second sets to train the model and the third set to evaluate its performance. Finally, we have created another architecture, an LSTM-CNN, to compare the performance of our model with that of the new one.