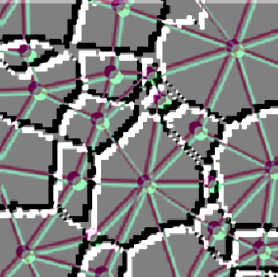
Machine learning helps understand the behavior of magnesium alloys
18-07-2023
Machine learning was used to map the distribution of dislocations in Mg alloys and predict their mechanical properties. A group of scientists from Finland, Spain, and NCBJ in Poland trained a deep learning model on a dataset of electron backscatter diffraction (EBSD) images of Mg alloys with different dislocation densities. This could lead to the development of novel materials with optimized mechanical properties. Their paper appeared in the July issue of Nature Scientific Reports..
Magnesium-based alloys (Mg alloys) are attractive for structural applications due to their low density and high strength-to-weight ratio. However, Mg alloys are also susceptible to a number of defects, primarily by dislocations, that alter their mechanical properties. Dislocations are massless defects in the crystal structure of a material and can cause the material to deform by moving through it. The distribution of dislocations in a material can have a significant impact on its mechanical properties, such as strength, ductility, and fatigue resistance.
“Traditional methods for mapping the distribution of dislocations in Mg alloys are time-consuming and labor-intensive,” explains Prof. Stefanos Papanicolau from Centre of Excellence NAMATEN at National Centre for Nuclear Research, Poland. “These methods typically involve using electron microscopy to image the dislocations in the material. However, electron microscopy is not a scalable method, and it can be difficult to obtain quantitative data on the dislocation density using this method.”
In the study, the scientists used machine learning to map the distribution of dislocations in Mg alloys and predict their mechanical properties. They used a deep learning algorithm to train a model on a dataset of images of Mg alloys with different dislocation densities. The model was then able to accurately predict the dislocation density in new images of Mg alloys. They also used the model to predict the mechanical properties of the Mg alloys, such as strength, ductility, and fatigue resistance.
FIG. 1. The supervised machine-learning workflow combining scanning electron microscope EBSD images of Mg alloys with graph neural net (GNN) based approach. The dislocation density maps obtained from mechanical deformation tests serve as the target data for the GNN model. The grain microstructure and associated attributes are utilized as relevant predictors. The combined data set is employed to construct a GNN, with each grain center location being a graph node, to be trained through the “encode-process-decode” architecture. The model can learn from underlying correlations between the grains’ microstructure and materials’ mechanical response and make predictions on the distribution of dislocations solely based on the former information. (Credits: Stefanos Papanicolaou and Karman Karimi / NOMATEN CoE, NCBJ)
The deep learning algorithm that was used is a convolutional neural network (CNN). CNNs are a type of deep learning algorithm well-suited for image processing tasks. CNNs are able to learn the spatial relationships between pixels in an image, which allows them to identify features in the image that are relevant to the task at hand. In this case, the task at hand was to identify dislocations in images of Mg alloys. The CNN was able to learn the spatial relationships between pixels in the images that were indicative of the presence of dislocations. The model was then able to use this knowledge to predict the dislocation density in new images of Mg alloys.
“The results show that machine learning can be used to accurately map the distribution of dislocations in Mg alloys and predict their mechanical properties,” stresses Prof. Papanicolau. “We were able to train a model that could accurately predict the dislocation density in new images of Mg alloys. We were also able to use the model to predict the mechanical properties of the Mg alloys, such as strength, ductility, and fatigue resistance.”
The results have a number of implications for the development of new Mg alloys. Mg alloys have excellent mechanical properties, but their microstructures are remarkably complex, with an intense interplay of a wide range of defects, including dislocations, twins, and grain boundaries. The development of complete databases that allow for the classification of possible microstructures can lead to novel materials with optimized mechanical properties. The current model shows that machine learning can be used to accurately map the distribution of dislocations in Mg alloys. This could lead to the development of new Mg alloys with improved mechanical properties by optimizing the distribution of dislocations in the material.
In future work, the authors plan to use machine learning to map the distribution of dislocations in Mg alloys with a wider range of compositions, loading and processing conditions, and their interplay with other types of defects, such as twin and grain boundaries.
The article was published in . Nature Scientific Reports on July, 2023.